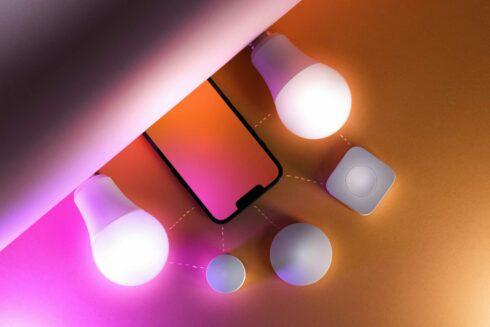
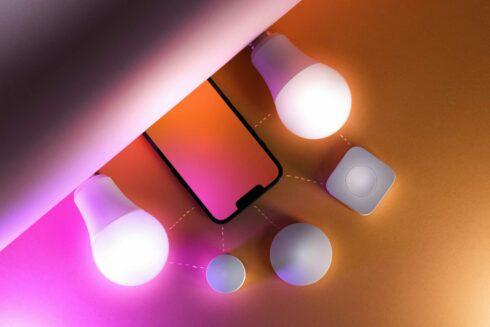
Time collection knowledge is a vital element of getting IoT units like good vehicles or medical tools that work correctly as a result of it’s gathering measurements primarily based on time values.
To study extra in regards to the essential position time collection knowledge performs in at this time’s linked world, we invited Evan Kaplan, CEO of InfluxData, onto our podcast to speak about this subject.
Right here is an edited and abridged model of that dialog:
What’s time collection knowledge?
It’s really pretty simple to know. It’s mainly the concept you’re gathering measurement or instrumentation primarily based on time values. The simplest method to consider it’s, say sensors, sensor analytics, or issues like that. Sensors may measure stress, quantity, temperature, humidity, gentle, and it’s normally recorded as a time primarily based measurement, a time stamp, if you’ll, each 30 seconds or each minute or each nanosecond. The thought is that you just’re instrumenting methods at scale, and so that you wish to watch how they carry out. One, to search for anomalies, however two, to coach future AI fashions and issues like that.
And in order that instrumentation stuff is completed, usually, with a time collection basis. Within the years passed by it may need been achieved on a common database, however more and more, due to the quantity of information that’s coming by way of and the actual time efficiency necessities, specialty databases have been constructed. A specialised database to deal with this form of stuff actually adjustments the sport for system architects constructing these refined actual time methods.
So let’s say you will have a sensor in a medical machine, and it’s simply throwing knowledge off, as you stated, quickly. Now, is it gathering all of it, or is it simply flagging what an anomaly comes alongside?
It’s each about knowledge in movement and knowledge at relaxation. So it’s gathering the information and there are some functions that we help, which might be billions of factors per second — assume a whole lot or 1000’s of sensors studying each 100 milliseconds. And we’re wanting on the knowledge because it’s being written, and it’s out there for being queried virtually immediately. There’s virtually zero time, nevertheless it’s a database, so it shops the information, it holds the information, and it’s able to long run analytics on the identical knowledge.
So storage, is {that a} large problem? If all this knowledge is being thrown off, and if there are not any anomalies, you might be gathering hours of information that nothing has modified?
Should you’re getting knowledge — some regulated industries require that you just maintain this knowledge round for a very lengthy time period — it’s actually necessary that you just’re skillful at compressing it. It’s additionally actually necessary that you just’re able to delivering an object storage format, which isn’t simple for a performance-based system, proper? And it’s additionally actually necessary that you just have the ability to downsample it. And downsample means we’re taking measurements each 10 milliseconds, however each 20 minutes, we wish to summarize that. We wish to downsample it to search for the sign that was in that 10 minute or 20 minute window. And we downsample it and evict a whole lot of knowledge and simply maintain the abstract knowledge. So it’s important to be excellent at that type of stuff. Most databases will not be good at eviction or downsampling, so it’s a very particular set of expertise that makes it extremely helpful, not simply us, however our rivals too.
We had been speaking about edge units and now synthetic intelligence coming into the image. So how does time collection knowledge increase these methods? Profit from these advances? Or how can they assist transfer issues alongside even additional?
I feel it’s fairly darn elementary. The idea of time collection knowledge has been round for a very long time. So in case you constructed a system 30 years in the past, it’s doubtless you constructed it on Oracle or Informatics or IBM Db2. The canonical instance is monetary Wall Avenue knowledge, the place you understand how shares are buying and selling one minute to the subsequent, one second to the subsequent. So it’s been round for a very very long time. However what’s new and completely different in regards to the house is we’re sensifying the bodily world at an extremely quick tempo. You talked about medical units, however good cities, public transportation, your vehicles, your house, your industrial factories, all the pieces’s getting sensored — I do know that’s not an actual phrase, however simple to know.
And so sensors communicate time collection. That’s their lingua franca. They communicate stress, quantity, humidity, temperature, no matter you’re measuring over time. And it seems, if you wish to construct a better system, an clever system, it has to start out with refined instrumentation. So I wish to have an excellent self-driving automobile, so I wish to have a really, very excessive decision image of what that automobile is doing and what that surroundings is doing across the automobile always. So I can practice a mannequin with all of the potential consciousness {that a} human driver or higher, may need sooner or later. So as to try this, I’ve to instrument. I then have to look at, after which must re-instrument, after which I’ve to look at. I run that technique of observing, correcting and re-instrumenting over and over 4 billion instances.
So what are among the issues that we’d stay up for when it comes to use circumstances? You talked about a couple of of them now with, you understand, cities and vehicles and issues like that. So what different areas are you seeing that this could additionally transfer into?
So to start with, the place we had been actually sturdy is power, aerospace, monetary buying and selling, community, telemetry. Our largest prospects are everyone from JPMorgan Chase to AT&T to Salesforce to quite a lot of stuff. So it’s a horizontal functionality, that instrumentation functionality.
I feel what’s actually necessary about our house, and changing into more and more related, is the position that point collection knowledge performs in AI, and actually the significance of understanding how methods behave. Primarily, what you’re attempting to do with AI is you’re attempting to say what occurred to coach your mannequin and what’s going to occur to get the solutions out of your mannequin and to get your system to carry out higher.
And so, “what occurred?” is our lingua franca, that’s a elementary factor we do, getting an excellent image of all the pieces that’s occurring round that sensor round that point, all that form of stuff, gathering excessive decision knowledge after which feeding that to coaching fashions the place individuals do refined machine studying or robotics coaching fashions after which to take motion primarily based on that knowledge. So with out that instrumentation knowledge, the AI stuff is mainly with out the foundational items, notably the actual world AI, not essentially speaking in regards to the generative LLMs, however I’m speaking about vehicles, robots, cities, factories, healthcare, that form of stuff.