The pharmaceutical trade operates below one of many highest failure charges of any enterprise sector. The success price for drug candidates getting into capital Section 1 trials—the earliest kind of scientific testing, which might take 6 to 7 years—is anyplace between 9% and 12%, relying on the yr, with prices to carry a drug from discovery to market starting from $1.5 billion to $2.5 billion, in response to Science.

This skewed steadiness sheet drives the pharmaceutical trade’s seek for machine studying (ML) and AI options. The trade lags behind many different sectors in digitization and adopting AI, however the price of failure—estimated at 60% of all R&D prices, in response to Drug Discovery Immediately—is a vital driver for corporations wanting to make use of expertise to get medication to market, says Vipin Gopal, former chief information and analytics officer at pharmaceutical large Eli Lilly, presently serving an analogous function at one other Fortune 20 firm.
“All of those medication fail as a result of sure causes—they don’t meet the standards that we anticipated them to satisfy alongside some factors in that scientific trial cycle,” he says. “What if we might determine them earlier, with out having to undergo a number of phases of scientific trials after which uncover, ‘Hey, that doesn’t work.’”
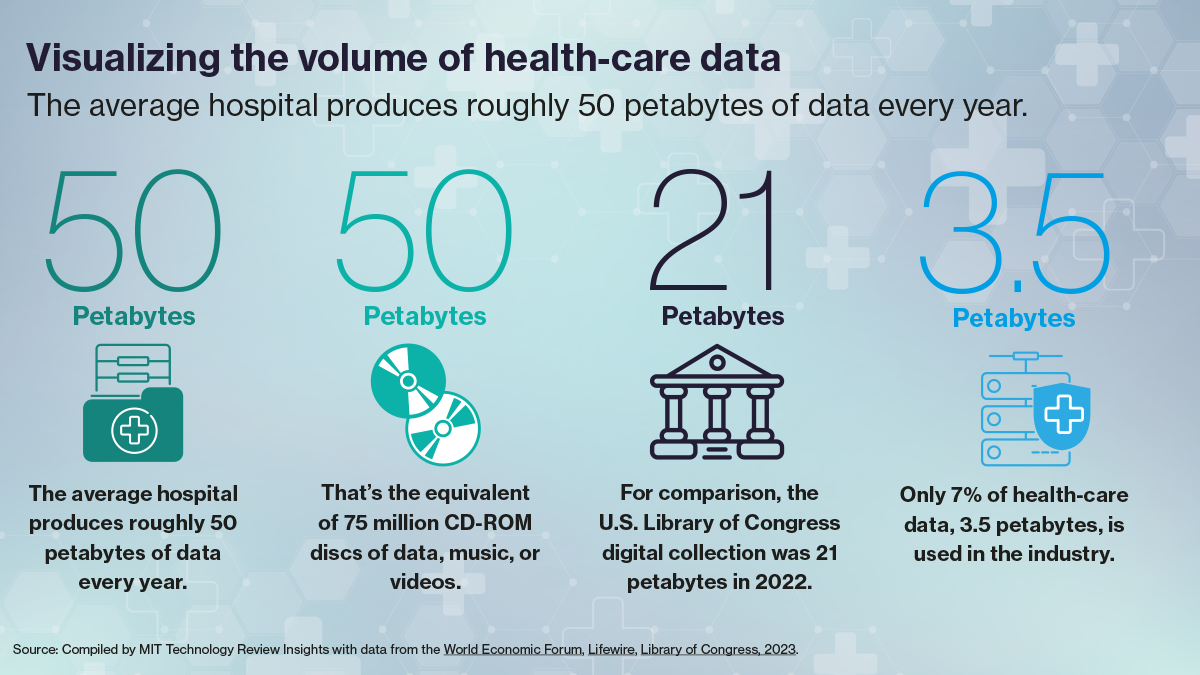
The pace and accuracy of AI can provide researchers the flexibility to shortly determine what’s going to work and what is not going to, Gopal says. “That’s the place the massive AI computational fashions might assist predict properties of molecules to a excessive degree of accuracy—to find molecules that may not in any other case be thought-about, and to weed out these molecules that, we’ve seen, finally don’t succeed,” he says.
This content material was produced by Insights, the customized content material arm of MIT Expertise Evaluation. It was not written by MIT Expertise Evaluation’s editorial workers.